I am currently a PhD student in deep learning. I am passionate about many fields of artificial intelligence, such as optimization, NLP, reinforcement learning…
My research is about frugal ML: I aim at making deep learning models more resource-efficient while maintaining their performance – the term “resource” referring for instance to time, memory, money, carbon emissions, etc. The PhD focuses mainly on transformers applied to NLP, as they are by far the most resource-consuming models in the field (hello ChatGPT). This is still a very broad topic, and multiple angles of attack are considered – from the architecture of the model to the optimization of the training process. A particular attention is given to adaptive models, i.e. models that can adapt their architecture to the task at hand dynamically, which is a promising way to make them more efficient and waste less resources.
PhD
December 2023 - today
PhD student in the MILES team of the LAMSADE lab, at Université Dauphine - PSL, under the supervision of Pr. Alexandre Allauzen. It is founded by the PEPR SHARP (Sharp Theoretical and Algorithmic Principles for frugal Machine Learning), which involves several actors collaborating towards frugal machine learning.
Title: Adaptive and Frugal Deep Learning Architectures
More details here.
Education
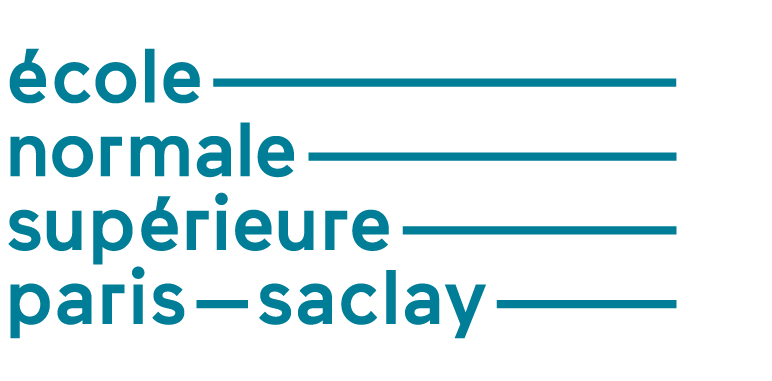
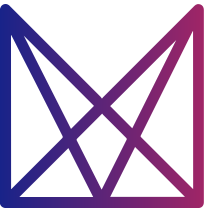
2022-2023: ENS Paris-Saclay
Master 2: Mathematics, Vision and Learning (MVA)
Selective Master's degree in machine learning, preparing the students for research. See the official website (in French), or here (in English).
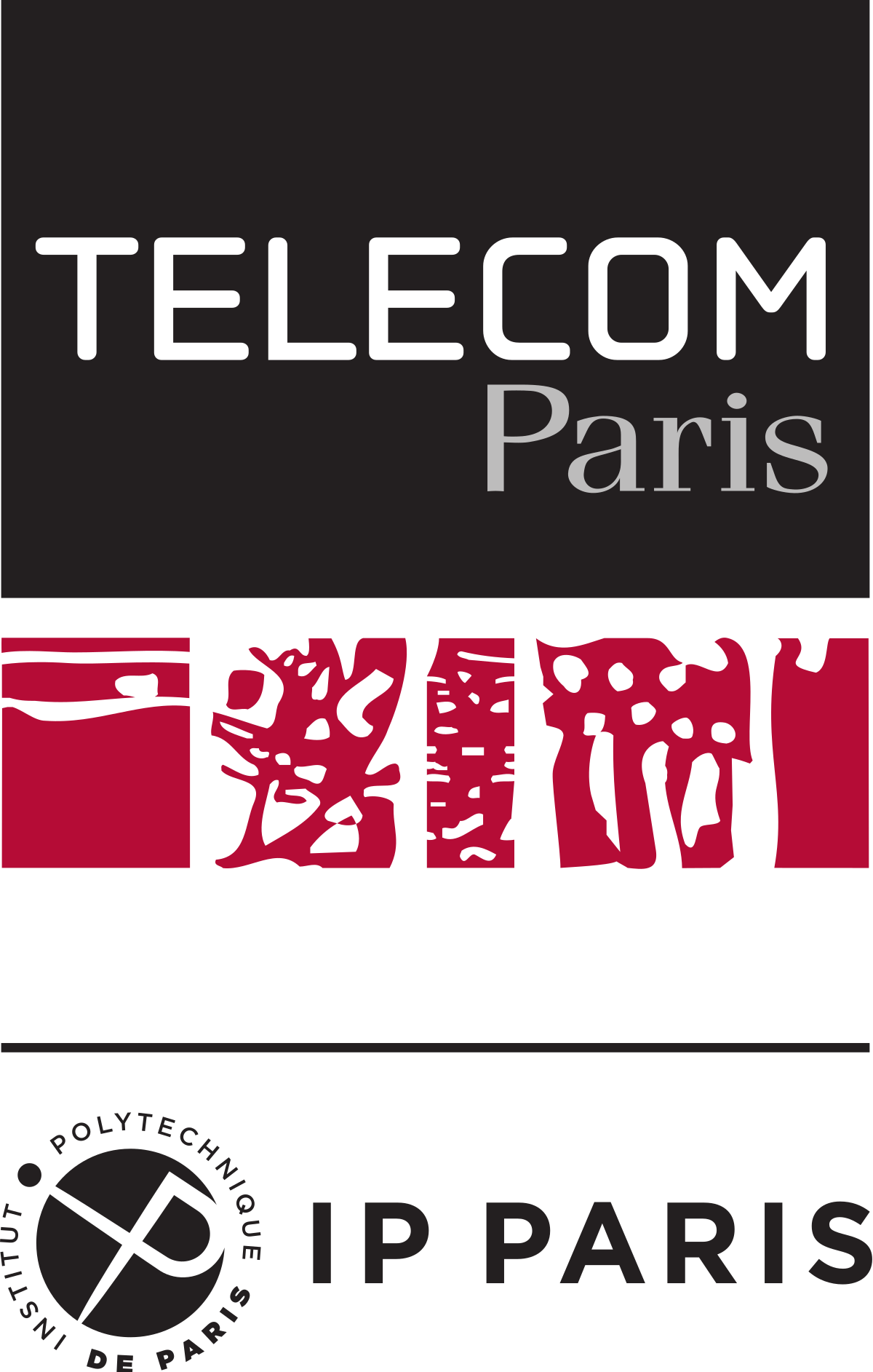
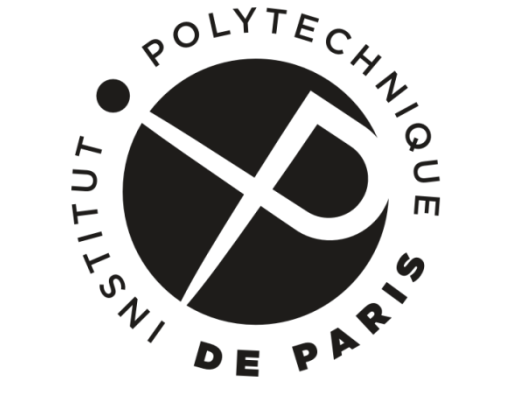
2020-2023: Télécom Paris
Master of Science
GPA: 4.0
3rd year: MVA Master at ENS Paris-Saclay
2nd year: SD (Data Science) and MITRO (Mathematics, Theoretical Computer Science and Operational Research) tracks